At OpenAI DevDay 2023, a groundbreaking advancement was revealed in the world of artificial intelligence. Among several innovations, the spotlight was on the GPT-4 Turbo Preview, a formidable addition to the GPT-4 family. This article delves into the features, capabilities, and implications of GPT-4 Turbo, setting a new benchmark in AI technology.
GPT-4 Turbo: A New Horizon
The GPT-4 Turbo Preview is not just an incremental update, but a substantial leap in the capabilities of AI language models. With a context window of 128k tokens, it stands head and shoulders above the existing GPT-4 models, which are limited to 8k and 32k tokens. This expansion isn't just about numbers; it represents a fundamental shift in how AI can process and interpret large volumes of data.
In practice, this means the ability to handle larger documents, maintain coherence over extended conversations, and provide more accurate and relevant responses in complex scenarios.
Understanding 'Lost in the Middle'
The introduction of GPT-4 Turbo, while a significant milestone, continues to face an intriguing challenge known as the "Lost in the Middle" phenomenon. This issue, identified through collaborative research by Stanford University, UC Berkeley, and Samaya AI, highlights a unique limitation in the realm of AI context processing.

The Core of the Challenge
In essence, "Lost in the Middle" refers to the difficulty AI models face in recalling information from the midsections of a large document. This phenomenon becomes more pronounced as the size of the context window increases. In models like GPT-4 32k and Anthropic Claude 100k, researchers observed a noticeable dip in efficiency when retrieving information from these middle sections, as compared to the beginning or end of the documents.
Implications for GPT-4 Turbo
GPT-4 Turbo, despite its advanced capabilities, is not immune to this challenge. The research conducted by Greg Kamradt has shed light on this issue within the new model. In his X post, Greg's findings reveal that GPT-4 Turbo's recall performance starts to degrade when handling more than 73k tokens.

This degradation ranges from 7% to 50% within the document's depth, indicating a significant variance in recall efficiency based on the location of information within the text.
This phenomenon poses a crucial balance for developers and users of GPT-4 Turbo. While the model can process an unprecedented amount of context, this capability must be tempered with the understanding that not all parts of the context are accessed equally. This can have important implications for how we structure information and queries when working with the model to ensure optimal performance.
GPT-4 vs. GPT-4 Turbo Performance
While "Lost in the Middle" presents a challenge, GPT-4 Turbo notably excels in its performance capabilities, especially when compared to its predecessor GPT-4. This is clearly illustrated in the research conducted by Shawn Wang, which provides valuable insights into the efficiency and effectiveness of GPT-4 Turbo.
Benchmarking Against GPT-4
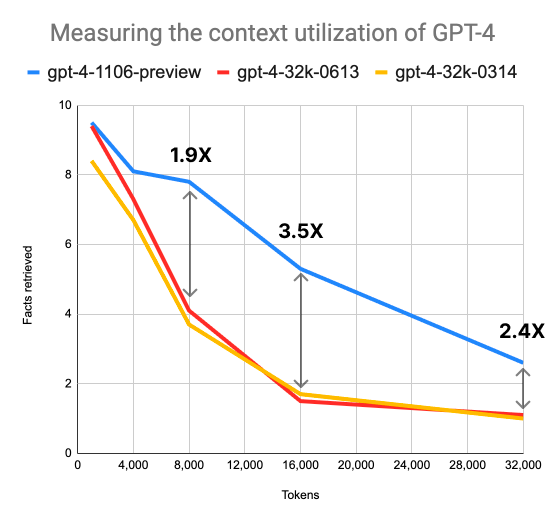
GPT-4 Turbo's enhanced context window significantly outperforms the standard GPT-4 in terms of context utilization. The comparison is striking: GPT-4 Turbo is found to be:
- 1.9 times more efficient with an 8k context window,
- 3.5 times with a 16k window,
- 2.4 times with a 32k window.
This improvement in performance is a testament to the advancements made in the model's ability to process and recall large amounts of information.
One of the most impressive aspects of GPT-4 Turbo is its ability to maintain high-quality performance even as the context window expands. Remarkably, the model's performance only degrades to the level of the standard GPT-4 when it reaches a 64k context window. This means that even at double the maximum context length of the standard GPT-4, Turbo maintains equivalent performance levels.
Current Limitations and Future Prospects
The Preview Phase
Currently, GPT-4 Turbo is in its preview phase, which means there are restrictions in place to manage its usage and ensure stability. These include rate limits for:
- 20 requests per minute and
- 100 requests per day.
While these limits might seem constraining, they are a standard part of the process for rolling out new technology responsibly. It allows for careful monitoring and tweaking as real-world usage data is collected.
A Path to Broader Accessibility
As GPT-4 Turbo progresses out of the preview phase, these rate limits are expected to be lifted. This transition will mark a significant step towards wider accessibility and application of the model. It opens up opportunities for more extensive testing and integration into larger-scale projects and platforms, unleashing the full potential of this advanced AI tool.
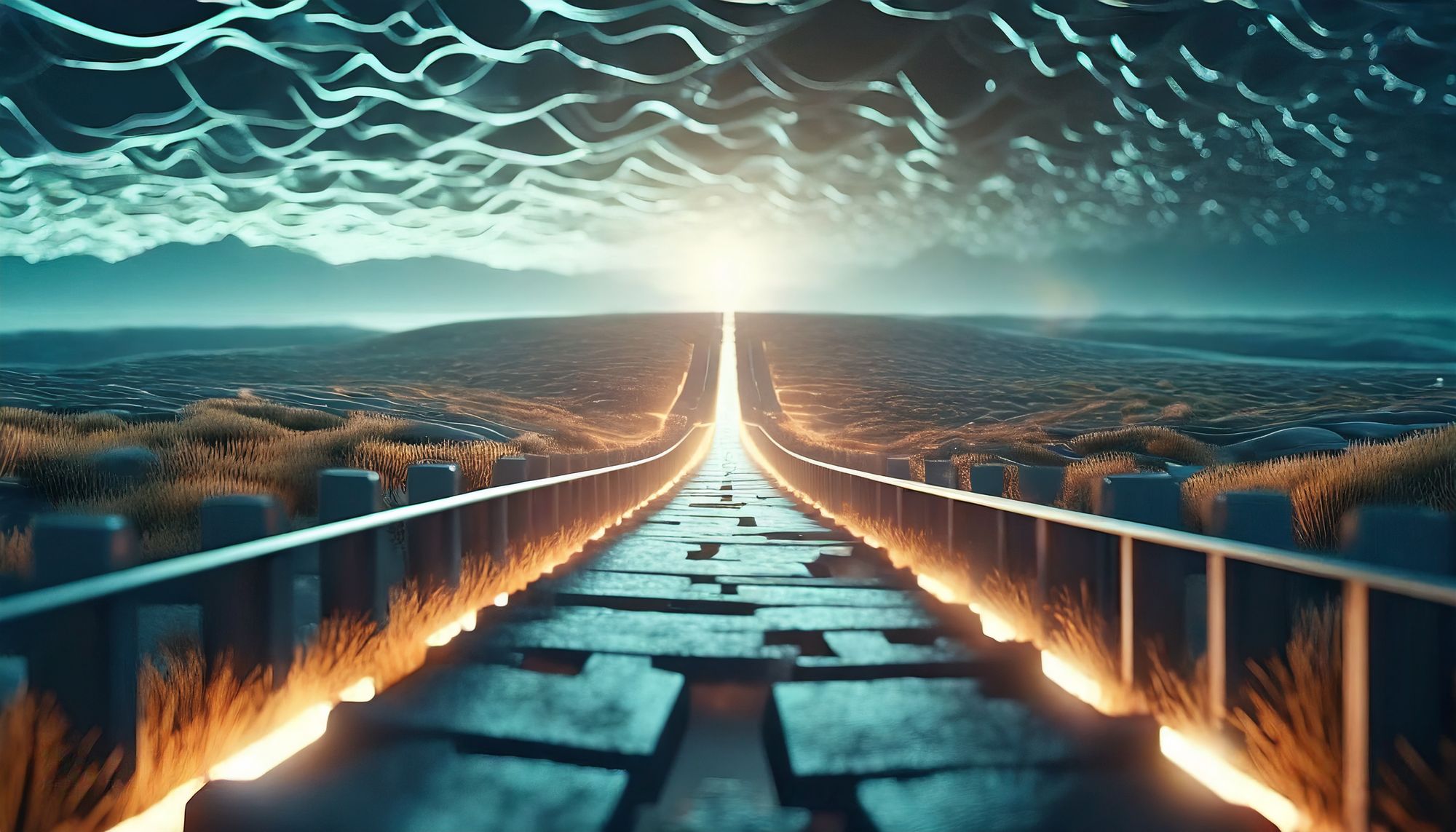
Closing Remarks
GPT-4 Turbo marks a significant leap in AI's capability to handle extensive context, offering improved performance over its predecessors.
While it still grapples with the "Lost in the Middle" phenomenon, its enhanced processing power opens up new avenues for complex problem-solving and integration into larger systems. As we witness these exciting developments, the future of AI looks more promising than ever.